Future-proofing your strategy with Generative AI guardrails
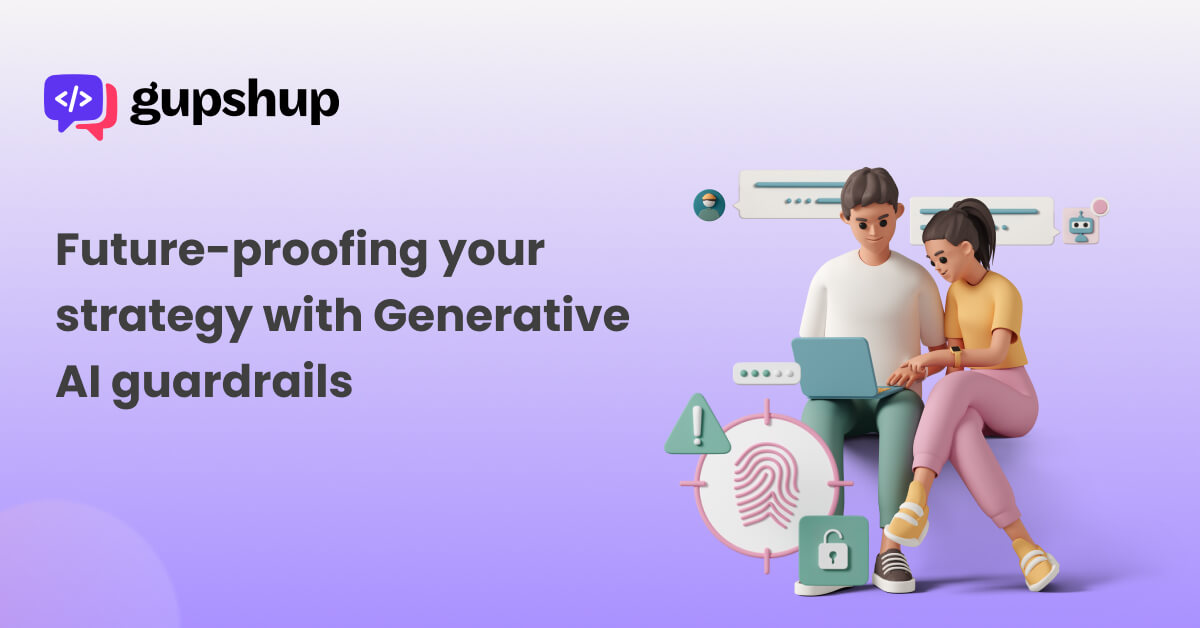
AI Guardrails – The How, What and Why, Answered
‘Guardrails’ -Unless one has been under a rock, hearing this word in almost every AI conversation since the launch of ChatGPT in 2022 has been inevitable. As a Business or IT leader looking to adopt Generative AI into your business, it is prudent to dig deeper beyond what Large Language Models or LLMs can do for you. In fact, this article will help you understand factors you need to consider before investing in or collaborating with an AI partner for customer or employee-led AI.
Generative AI, as we’ve seen, has multiple applications for enterprises spanning text, code, voice, and image generation, summarization, query answering and more, allowing brands to automate a majority of their business communications with AI-enabled virtual assistants.
But what happens when your virtual assistants step outside of what your enterprise deems accurate or ethically sound responses and provides a reply riddled with bias, or one that’s simply untrue? That’s exactly why ‘Guardrails’ in Generative AI is such a trending topic within the technology community – who decides where the AI is seeking its responses from? Is it within the context of your brand? Does it have controls in place to prevent the leakage of sensitive data? Can it share the source of information? Does it steer the conversation to achieve the intended objective?
Read on to learn what Guardrails comprise, what putting them into action looks like, and most importantly why your decision on an AI partner can make or break your customer and employee experience.
The 101 on Guardrails in Generative AI
As you may know, most open source Large Language Models (LLMs) are not ready to handle enterprise usage from the get-go. They rely heavily on the processes of fine-tuning and prompt engineering to get the source data and AI trained to a level where they can independently automate an enterprise’s communications. Finetuning as a process involves training the LLM on domain-specific data sources for it to be deemed fit for a specific industry, vertical, or use case. Prompt engineering plays a crucial role by providing concise and pointed instructions to the AI models, hence impacting the type of response generated.
Here is where it gets interesting! How do we validate if base data sources are secure? Are the LLMs also going to be interacting with external data in the process of handling queries? Is this finetuned data set handled securely to ensure compliance with privacy regulations?
Here is where the role of Guardrails arises – simply put, they are controls put in place to provide assurance of safety, appropriateness, and unbiased, accurate replies to user queries.
The state of Generative AI and LLMs…
Generative AI as Gartner’s Hype Cycle for Artificial Intelligence puts it, is going through its peak of inflated expectations -as cited in its press release, “Generative artificial intelligence (AI) is positioned on the Peak of Inflated Expectations on the Gartner, Inc. Hype Cycle for Emerging Technologies, 2023, projected to reach transformational benefit within two to five years. Generative AI is encompassed within the broader theme of emergent AI, a key trend on this Hype Cycle that is creating new opportunities for innovation.”
“The popularity of many new AI techniques will have a profound impact on business and society,” said Arun Chandrasekaran, Distinguished VP Analyst at Gartner. “The massive pretraining and scale of AI foundation models, viral adoption of conversational agents and the proliferation of generative AI applications are heralding a new wave of workforce productivity and machine creativity.”
Source: Gartner
In short, the AI enablers, providers, and inventors that will survive are those looking at the utilization of this technology from a future-forward lens.
Ways to mitigate risks with Guardrails for Generative AI
- Deploying LLMs finetuned to industries or domains: The process of finetuning to a specific industry allows defining of the quality and validity of relevant data sources that the AI is trained on, allowing for a far richer and more accurate set of responses meted out by the end platform or chatbot. This keeps the source of data that the model seeks answers from a limited domain-specific set of sources, which greatly reduces the possibilities of bias, inaccuracies, and inappropriate responses.
- Ensuring regulatory and enterprise compliance: With these controls in place, enterprises can ensure that compliance related to regulatory laws or information security are met adequately, while also adding controls specific to the enterprise’s own policies. An advanced system of guardrails would allow stricter controls to be stricter in certain topics than others, we call this ‘teach mode’.
- Continuous human-driven QA: The process of training, launching, and maintaining a generative AI bot powered by LLMs relies heavily on the expertise of the team running the QA and testing. They stay on top of the performance and isolate any loopholes or gaps in the performance of an LLM. Continual finetuning and data-driven enhancements have to be undertaken for optimal output.
- Hallucination controls: While 100% elimination of hallucinations from LLMs generated output is still a while away, there are several boundaries that can be defined that reduce the possibility of responses that seem sensible, but in reality are false or made up.
Key considerations while setting up Guardrails for Generative AI
To launch a fully automated and trusted source of business-to-consumer or business-to-employee engagement platform supported by LLMs, having clear outcomes or goals in mind is key. Clarity on the type of use cases of the stage of the funnel you see your Gen AI bot supporting users across, and the sensitivity of data the bot would be handling are some of the key considerations while building generative capabilities that users can learn to rely on and trust.
- Explainability: Another Guardrail layer is that of providing the cited sources of information that support the response.
- Nuanced control: One size does not fit all! System-wide strict guardrails settings would reduce the creativity of the model output and make the user interactions less natural. Organizations should consider identifying areas and topics that are critical and employ stricter guardrail settings in those areas.
- Guardrails for the LLM: Not all LLMs are made equal, each one has its strengths and weaknesses. As each LLM responds differently to different kinds of guardrails, it becomes important to have the option of choosing different LLMs.
In conclusion…
Successful generative AI deployments at an enterprise scale are not limited to the quality of the output, but rather, output that’s reliable, in line with responsible AI principles, with controls in place to address issues of compliance, bias, hallucinations, and relevancy.
The ‘core’ of building and launching a Generative AI bot, whether voice or text-based, lies in its ability to meet enterprise requirements in accordance with AI Governance policies. As controls within the AI space become more formalized over time, adopters who already have the basics in place will be leaps and bounds ahead of those looking to be first-to-launch in the market with poorly tested platforms.
Stay ahead and go Guardrail-first in your enterprise deployments. To learn more about how Gupshup’s ACE GPT ensures safety, accuracy, and quality performance for your industry, book a sales consultation today https://www.gupshup.io/contact-us